EE 655000 Machine Learning 機器學習
Spring 2023, Mon. 17:30 to 20:20 , Location DELTA台達 215
Instructor: Min Sun
TAs: 蔡馥任 qwe846132@gapp.nthu.edu.tw
吳京軒 justinwu880520@gmail.com
李明峯 li871030@gmail.com
邵柏翔 b99872599@gmail.com
張君豪 howard88315@gapp.nthu.edu.tw
Suraj Dengale surajdengale@gapp.nthu.edu.tw
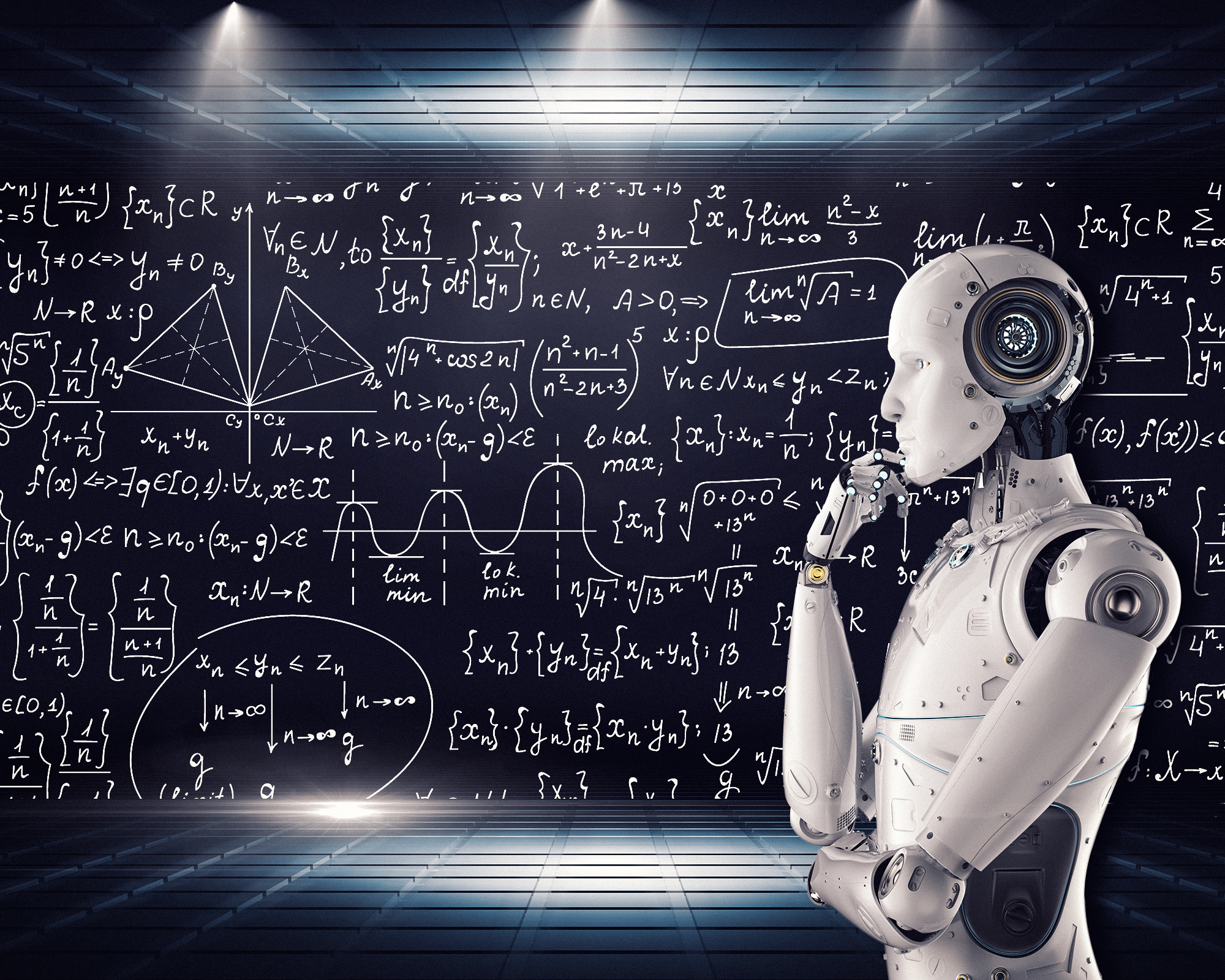
Course Description
機器學習領域有各種不同演算法,讓電腦可以從資料中學習,完成目標任務。隨著各領域數位化資料的累積,機器學習已經應用在五花八門 的各種領域。例如早期應用在資料探開,搜索,推薦上。到後來可以應用在各種非結構資料上,如語音、影像、文字等。如今機器學習更是 應用在各種科學領域,例如天文學、生物學、化學等。 機器學習結合了統計、數學與資訊科學等學門。廣義來說,機器學習研究如何讓電腦具有學習的能力,從以往的經驗及 數據中學習到知 識,以增進電腦本身的效能,因此機器學習也可解釋為利用資料來建立一些模擬真實世界的模型 (Models),利用這些模式來描述資料中 的特徵(Patterns)以及關係(Relations)。這些模式有兩種用處,第 一,瞭解資料的特徵與關係可以提供決策所需要的資訊。第 二,資料的特徵可以幫助進行預測。
本課程包括三份手寫作業以及三份程式作業,以及自學ML應用介紹(ML self-tutorial)、期中/期末報告 (最多3人一組)。 請在後面了解自學ML應用介紹(ML self-tutorial)、期中/期末報告等要求。syllabus.
Prerequisites
本課程需要使用Python編寫程式,此外會使用到線性代數、微積分、機率等概念。Textbook
Christopher M. Bishop, Pattern Recognition and Machine Learning, Springer, 2006, ISBN: 0387310738Resource
Machine Learning by Prof. Andrew NgGrading
總成績保括- 60% 三份手寫作業以及三份程式作業。
- 35% 期中簡報、期末簡報、期末報告. 最多3人一組。
- 5% 自學ML應用介紹(ML self-tutorial)。
Important Links
Contact Info and Office Hours
教授聯繫資訊如下。- Min Sun: sunmin@ee.nthu.edu.tw
- Min Sun, Delta 962, Tel: 035731058, Time: Mon. 15:30 - 16:30
- TAs, EECS Building 711 & 804, Time: Mon. 15:00 - 17:00
Tentative Syllabus
Week | Class Dates | Topic | Slides | Recording | Extra Info (e.g., Homework/Exam) |
---|---|---|---|---|---|
1 | M, Feb. 13 | Opening | pdf pdf pdf | Group Form Out | |
Math Review | |||||
Python tutorial | |||||
2 | M, Feb. 20 | Intro. to ML | pdf pdf | Homework 1 out | |
Probability Distributions | |||||
3 | M, Feb. 27 | Holiday (2/28) | Group Finalisation (3/1) | ||
4 | M, Mar. 6 (No Physical Class) | Probability Distributions | link | Class Recorded on EEclass | |
Linear Regression Models | |||||
Intro to Competitions | link | ||||
5 | M, Mar. 13 | Linear Classification Models | Homework 2 out | ||
Self-Tutorial | |||||
6 | M, Mar. 20 | NN | |||
PyTorch Intro | Colab | ||||
7 | M, Mar. 27 | NN | Homework 1 due | ||
Self-Tutorial | |||||
8 | M, Apr. 3 | April Holiday | |||
9 | M, Apr. 10 | Mid-Term Presentations | Each team present 3-5 minutes. Homework 3 out | ||
10 | M, Apr. 17 | Kernel Methods | Homework 2 due | ||
Self-Tutorial | |||||
11 | M, Apr. 24 | Sparse Kernel Machines | |||
Self-Tutorial | |||||
12 | M, May. 1 | Graphical Model | |||
13 | M, May. 8 | Mixture Models and EM | |||
Sampling Methods | |||||
Self-Tutorial | |||||
14 | M, May. 15 | Ensemble Learning | |||
Buffer | |||||
15 | M, May. 22 | Competiton Week: Open to team-based meeting | Competition due Firday mid-night. Homework 3 due |
||
16 | M, May. 29 | Final presentation | <<<<<<< HEAD | Top three winner each present 10 minutes. Others present 3 minutes. |
=======
>>>>>>> f8b706685b097825dc2abea20a813bcac4d42292
|
17 | M, June. 5 | No Class | Final Report Due |